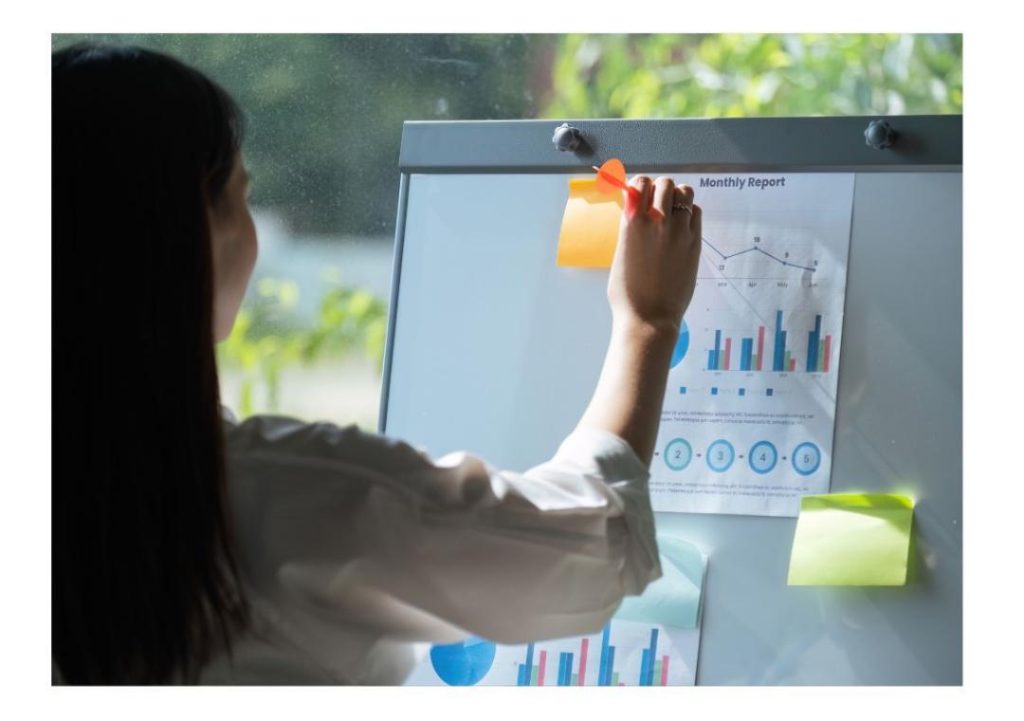
Do your systems deliver performance or just track it?
In today’s AI-driven world, performance is no longer just an aspiration, but an output of the operating model. This is especially true for AI companies, where models optimize daily, dashboards update hourly, and everything is measurable, tweakable, and designed to improve without manual push. This has created a culture of constant progress, where performance is no longer a goal, but a byproduct of the system itself.
The traditional approach to performance tracking is often manual, ad-hoc, and reactive. It involves setting goals, tracking progress, and making adjustments based on feedback. However, this approach is limited in its ability to drive continuous improvement, as it relies on human intervention to identify areas for improvement.
In contrast, AI companies have adopted a more proactive and automated approach to performance tracking. By integrating performance metrics directly into their systems, they are able to optimize their models, dashboards, and processes in real-time, without the need for manual intervention. This has enabled them to achieve a level of performance that was previously unimaginable.
So, how do AI companies achieve this level of performance? The key lies in their operating model, which is designed to deliver performance rather than just track it. Here are some key principles that underpin this approach:
-
Integrate performance metrics: AI companies integrate performance metrics directly into their systems, allowing them to track progress in real-time. This enables them to identify areas for improvement and make adjustments accordingly.
-
Automate optimization: By automating the optimization process, AI companies are able to optimize their models, dashboards, and processes in real-time, without the need for manual intervention. This enables them to achieve a level of performance that was previously unimaginable.
-
Design for continuous improvement: AI companies design their systems with continuous improvement in mind. This involves setting goals, tracking progress, and making adjustments based on feedback. However, this approach is more proactive and automated, rather than reactive and manual.
-
Use data to drive decisions: AI companies use data to drive their decision-making process. This involves analyzing performance metrics to identify areas for improvement and making adjustments accordingly.
-
Foster a culture of experimentation: AI companies foster a culture of experimentation, where employees are encouraged to try new things and take risks. This enables them to identify new areas for improvement and develop innovative solutions.
In conclusion, AI companies have achieved a level of performance that was previously unimaginable by integrating performance metrics directly into their systems, automating optimization, designing for continuous improvement, using data to drive decisions, and fostering a culture of experimentation. This has created a culture of constant progress, where performance is no longer a goal, but a byproduct of the system itself.
Source: https://www.growthjockey.com/blogs/ai-companies-performance-breakthrough